Machine learning sheds new light on brain connectomes by predicting whether neurons inhibit, excite, or otherwise modulate each other
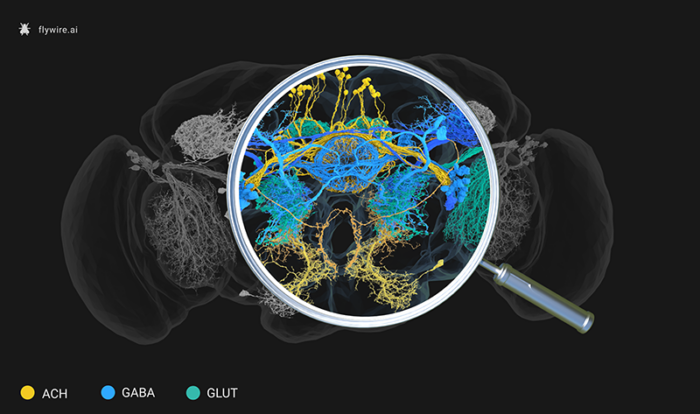
Over recent years, neuroscientists have been building brain ‘connectomes’ – maps in which all neurons and the synaptic connections between them can be visualised. Now Greg Jefferis’ group in the LMB’s Neurobiology Division in collaboration with scientists at the Janelia Research Campus, have predicted the ‘sign’ of each synaptic connection in the whole fruit fly (Drosophila melanogaster) brain. They have used machine learning to know whether a neuron inhibits, excites, or otherwise modulates its partners.
Neurons communicate with each other by secreting ‘neurotransmitters’, chemical messengers that they release at synapses to change the electrical activity in a downstream cell. By comparing neurons in the available connectomes with experimental data, the group identified hundreds of neurons that used the main neurotransmitters. Alexander Bates, a former Ph.D. student at the LMB now at Harvard Medical School, and Philipp Schlegel, another member of Greg’s group, did the biological analysis and built ‘ground truth’ data, which accounted for less than 2% of cells in the brain.
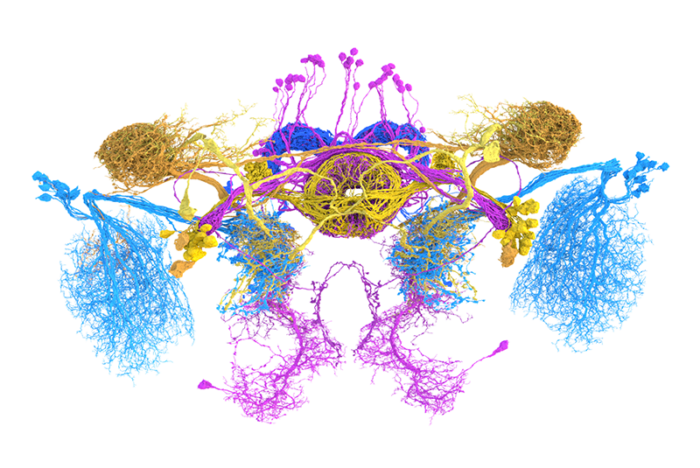
Nils Eckstein and Jan Funke at the Janelia Research Campus used hundreds of thousands of synapses from this small set of neurons, to build a machine learning model. The model was able to learn the image features that differentiated six different types of neurotransmitters (acetylcholine, GABA, glutamate, dopamine, serotonin, or octopamine – the insect version of noradrenaline) and predict these labels across the rest of the dataset.
Their approach was successful, getting 94% of known neurons’ neurotransmission correct – which is surprising as previously it was not possible for researchers to tell the difference between, for example, a synapse that releases acetylcholine (excitatory) and one that releases GABA (inhibitory). The research team at the Janelia Research Campus devised a method to work out what the computer was identifying by iteratively mutating pixels in images of synapses until the trained network predicted the wrong label. Using this approach, they could deduce which image features are the most important. In the case of acetylcholine and GABA, it was the brightness of the synaptic ‘gap’ between two connected neurons that proved key. These differences were then successfully confirmed in independent synapse data, so machine learning was able to identify features that humans had ignored until now.
This study is a significant step forward in increasing the interpretability and usability of connectomic data sets. It will help develop better hypotheses for brain function and has already been used in unpublished work simulating large parts of the fly brain. Their results also show that neurons in the brain that are known to be born together, tend to express the same neurotransmitter, revealing a general developmental principle about how the brain is built from its stem cells. Finally, this work also provides a powerful demonstration of how AI can be used to extract molecular information from high resolution images of cells – this is a space that is likely to see significant advances in the next few years.
This work was funded by UKRI MRC, Wellcome Trust, National Institutes of Health, ERC, EMBO, Boehringer Ingelheim Fonds, the Howard Hughes Medical Institute, and Neuronex2 programme (MRC/National Science Foundation). Additional participants include the Drosophila Connectomics Group at the Department of Zoology, and Prof. Volker Hartenstein at UCLA.
Further references
Neurotransmitter Classification from Electron Microscopy Images at Synaptic Sites in Drosophila melanogaster. Eckstein, N., Bates, AS., Champion, A., Du, M., Yin, Y., Schlegel, P., Lu, AKY., Rymer, T., Finley-May, S., Paterson, T., Parekh, R., Dorkenwald, S., Matsliah, A., Yu, SC., McKellar, C., Sterling, A., Eichler, K., Costa, M., Seung, S., Murthy, M., Hartenstein, V., Jefferis, GSXE., Funke, J. Cell.
Greg’s group page
Previous Insight on Research articles
How flies use smell to determine the identity and position of other flies
A map to show the way to sense smell, temperature, and humidity