New cryo-EM imaging software incorporates cutting-edge algorithms and neural networks to remedy a long-standing problem in structural biology and enables the identification of unknown components
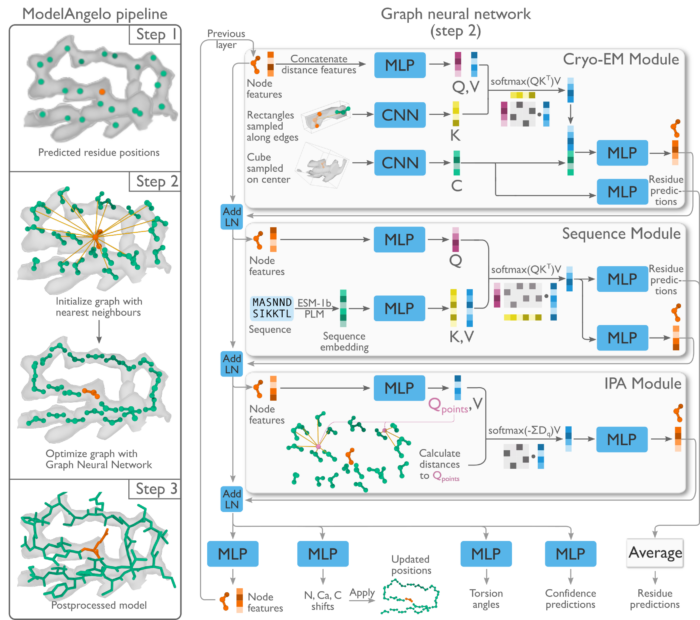
Electron cryo-microscopy (cryo-EM) is a crucial tool for determining biological structures, which in turn, allow researchers to advance understanding of the molecular processes which underpin life. Once experimental images are captured, software such as RELION can be used to reconstruct density maps of the imaged biomolecules to create three-dimensional volumes. However, to achieve an atomic structure, experienced researchers must spend a considerable amount of time manually interpreting the densities in three-dimensional computer graphics programmes. To overcome this, Kiarash Jamali, a Ph.D. student in Sjors Scheres’ group in the LMB’s Structural Studies Division, has developed the programme ModelAngelo, which is not only capable of automated model building at atomic levels, but has also shown aptitude at identifying novel proteins.
The neural networks behind ModelAngelo are based on the same fundamental building blocks as those used by ChatGPT and AlphaFold. However, these previous machine learning approaches were able to utilise anywhere between hundreds of thousands to tens of millions of pieces of training data. In contrast, there are fewer than 13,000 cryo-EM structures which have been solved to resolutions greater than 4Å. To overcome this limitation, ModelAngelo was designed to use a multi-modal, machine-learning approach, which incorporates three different data inputs, each in different forms; three-dimensional volume cryo-EM maps, text indicating the amino acid sequences of the proteins in the sample, and graphs of the intermediate atomic model built at each step.
Using these specialist neural networks, ModelAngelo is able to produce models of a comparable quality to expert structuralists manually interpreting results in a fraction of the time. The programme has also demonstrated success in modelling nucleotide backbones.
To illustrate the advances presented by ModelAngelo, it needed just a few hours to build complex subunit proteins that perform photosynthesis in algae to a high degree of completion (the supercomplex of the phycobilisome and transmembrane light-harvesting complexes). This large complex containing over 150,000 residues in 81 unique protein chains previously took months of work for researchers to manually build the atomic model.
Beyond the benefit of saving huge amounts of time in structural studies, ModelAngelo has shown further use with its capabilities to identify novel proteins which were previously unknown to researchers using the cryo-EM map alone. To achieve this, Lukas Käll at the KTH Royal Institute of Technology in Stockholm, Sweden, further developed the software, implementing a database searching algorithm using hidden Markov Models. Again studying the above supercomplex, ModelAngelo was able to identify six novel protein chains that prior to this were unknown, despite extensive manual effort when mapping the structure.
ModelAngelo is a transformative addition to the toolkit of structural biology. It promises to drastically reduce the time needed for atomic structure determination, and has proved itself more capable than humans in identifying proteins with unknown sequences. Already, it is in use in several different projects, including in drug discovery pipelines in pharmaceutical companies. Aside from its applicable uses, the use of such divergent data sources represents a significant advancement in the field of machine learning, and has the potential to be further developed and implemented in a vast range of scenarios.
For his work designing and implementing ModelAngelo, Kiarash received the 2023 Perutz Student Prize.
This work was funded by UKRI MRC, EU Horizon 2020, the National Institutes of Health and the Knut and Alice Wallenberg Foundation.
Further references
Automated model building and protein identification in cryo-EM maps. Jamali, K., Käll, L., Zhang, R., Brown, A., Kimanius, D., and Scheres, SHW. Nature
Sjors’ group page
Lukas Käll’s page