The Nomaly system is a genetic outlier detector, capable of making knowledge-based interpretations for phenotype variants at the organism and cellular level
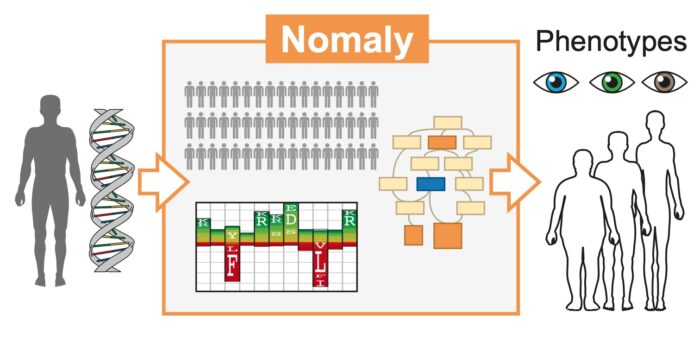
In recent years, due to the expansion of technologies that produce human genomic data, the volume of data available has hugely increased. However, innovation in methods for genome interpretation is urgently required to deliver on the growing promise of genomics. With appropriate tools for deciphering an individual’s genetic variation, it could be possible to predict potential disease risks or organism-level phenotypes (observable characteristics or traits including morphology, developmental processes, and biochemical and physiological properties). Julian Gough’s group in the LMB’s Structural Studies Division has devised a new, computational method for genome analysis. The new genetics-first approach is capable of making knowledge-based phenotype interpretations for exome-wide genetic variants at the organism and cellular level.
This new method – the Nomaly system – is designed to be a genetic outlier detector, working on the premise that an extreme genetic outlier is indicative of an outlier in phenotype. It uses a predictive ab initio approach, identifying the genetic outliers as the starting point of analysis, rather than beginning at the chosen phenotype and working backwards. Where previous methods have asked, ‘Does the data contain the answer to my question?’ the Nomaly system instead asks, ‘The answers to which questions lie within these data?’
Chang Lu, a former postdoc in Julian’s group, validated the new method against three independent cohorts. For the principal cohort, the group recruited people from across the world who were in possession of their personal direct-to-consumer genotype data and asked them to submit their genome for analysis and then respond to a phenotype questionnaire. The group also used an established dataset from children with developmental disorders and another from a cell bank with hundreds of people’s stem cells and corresponding genome sequences. For the latter dataset, the group collaborated with Miguel Bernabe-Rubio, James Williams and Davide Danovi at the Centre for Gene Therapy and Regenerative Medicine at King’s College London who conducted the supporting experimental work.
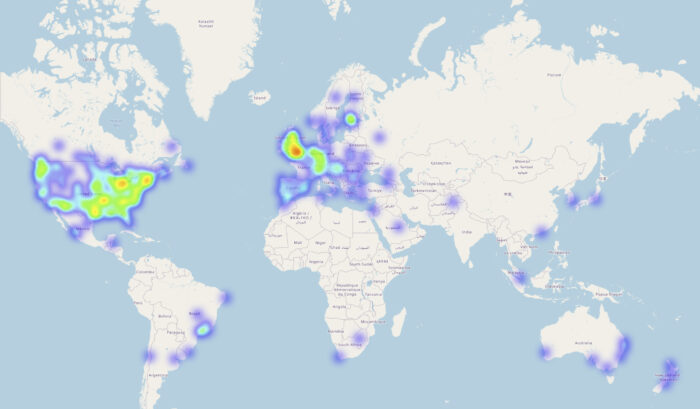
The work deployed a model based on protein domains, which are the functional units of proteins. Hidden Markov models built on protein domains enabled the quantification of structural and functional effects of variants, and this was linked to different phenotypes identified in the cohort. The group identified plausible genetic causes for 40 phenotypes such as nail dysplasia and abnormal mitral valve morphology.
This method is a substantial addition to the field of genome analysis. In an era where millions of people’s genomes have now been sequenced, continual revolution in computational methods is needed to enable researchers to extract important biological information, and ultimately medical value, from this resource. The method can be further applied for medical discovery and, when used alongside other derivative methods, may contribute to advancements in personalised medicine.
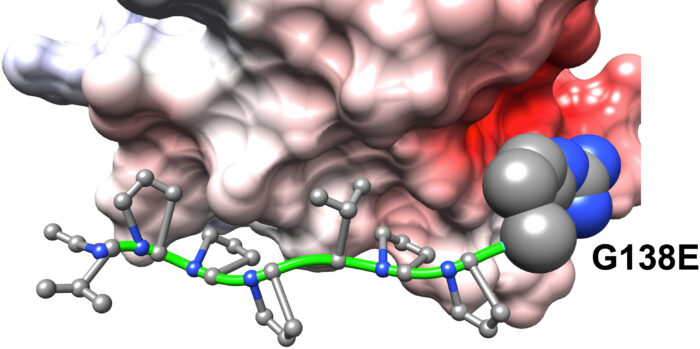
This research was largely facilitated by the ‘donation’ of personal genome data to the group’s online study. The genomics study is still open for those who are in possession of their personal direct-to-consumer genotype data and wish to contribute to the advancement of science and medicine. Another option for those wishing to contribute is openSNP, created by Bastian Greshake Tzovaras, who also contributed to this study.
This work was funded by UKRI MRC, UKRI BBSRC, the Wellcome Trust and the Center for Research and Interdisciplinarity (CRI).
Further references
Hypothesis-free phenotype prediction within a genetics-first framework. Lu, C., Zaucha, J., Gam, R., Fang, H., Smithers, B., Oates, ME., Bernabe-Rubio, M., Williams, J., Zelenka, N., Pandurangan, AP., Tandon, H., Shihab, H., Kalaivani, R., Sung, M., Sardar, AJ., Tzovoras, BG., Danovi, D., Gough, J. Nature Communications
Julian Gough’s group page
Centre for Gene Therapy and Regenerative Medicine, King’s College London