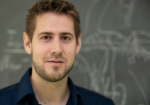
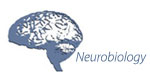
How do animals store learned behaviours in their neuronal networks and retrieve them when performing those behaviours? It is widely believed that the connections between neurons, or synapses, are the memory substrate. The sum total of all these synaptic connections is called the connectome.
Using the zebra finch songbird as a model, we investigate how song memories are stored and retrieved from the underlying brain circuits. These birds can perform songs as an adult that they have practiced as a juvenile, not unlike how humans learn language.
To map these brain circuits at sufficient resolution to see synapses, we employ high-throughput 3D electron microscopy. Vast amounts of image data result from this process, far more data than someone could ever look through manually. Thus, to analyse the data, we employ state-of-the-art deep learning techniques to infer the connectomic map, and let the artificial neural networks reconstruct the real ones.
Our long-term goal is to mechanistically understand how a learned behaviour, the zebra finch song, is encoded in the underlying synaptic wiring patterns, and create a link between the specific behaviour of an individual and the connectome it is based on.
Fully funded Phd and Postdoc positions available
Selected Papers
- Schubert,P.J., Saxena, R., Kornfeld, J. (2024)
DeepFocus: Fast focus and astigmatism correction for electron microscopy
Nature Communications 15(1): 948 - Sigmund, F., Berezin, O., Beliakova, S., Magerl, B., Drawitsch, M., Piovesan, A., Gonçalves, F., Bodea, S., Winkler, S., Bousraou, Z., Grosshauser, M., Samara, E., Pujol-Martí, J., Schädler, S., So, C., Irsen, S., Walch, A., Kofler, F., Piraud, M., Kornfeld, J., Briggman, K., Westmeyer, G.G. (2023)
Genetically encoded barcodes for correlative volume electron microscopy
Nature Biotechnology 41(12): 1734-1745 - Schubert, P.J., Dorkenwald, S., Januszewski, M., Klimesch, J., Svara, F., Mancu, A., Ahmad, H., Fee, M.S., Jain, V., Kornfeld, J. (2022)
SyConn2: dense synaptic connectivity inference for volume electron microscopy
Nature Methods 19(11): 1367-1370 - Svara, F., Förster, D., Kubo, F., Januszewski, M., Dal Maschio, M., Schubert, P.J., Kornfeld, J., Wanner, A.A., Laurell, E., Denk, W., Baier, H. (2022)
Automated synapse-level reconstruction of neural circuits in the larval zebrafish brain
Nature Methods 19(11): 1357-1366 - Egger, R., Tupikov, Y., Elmaleh, M., A Katlowitz, K., E Benezra, S., Picardo, M.A., Moll, F., Kornfeld, J., Jin, D.Z., Long, M.A. (2020)
Local axonal conduction shapes the spatiotemporal properties of neural sequences
Cell 183(2): 537-548. e12 - Schubert, P.J., Dorkenwald, S., Januszewski, M., Jain, V., Kornfeld, J. (2019)
Learning cellular morphology with neural networks
Nature communications 10(1): 2736 - Kornfeld, J., Denk, W. (2018)
Progress and remaining challenges in high-throughput volume electron microscopy
Current opinion in neurobiology 50: 261-267 - Januszewski, M., Kornfeld, J., Li, P.H., Pope, A., Blakely, T., Lindsey, L., Maitin-Shepard, J., Tyka, M., Denk, W., Jain, V. (2018)
High-precision automated reconstruction of neurons with flood-filling networks
Nature Methods 15(8): 605-610 - Kornfeld, J., Benezra, S.E., Narayanan, R.T., Svara, F., Egger, R., Oberlaender, M., Denk, W., A Long, M. (2017)
EM connectomics reveals axonal target variation in a sequence-generating network
Elife 6: e24364 - Dorkenwald, S., Schubert, P.J., Killinger, M.F., Urban, G., Mikula, S., Svara, F., Kornfeld, J. (2017)
Automated synaptic connectivity inference for volume electron microscopy
Nature Methods 14(4): 435-442 - Giret, N., Kornfeld, J., Ganguli, S., Hahnloser, R.H.R. (2014)
Evidence for a causal inverse model in an avian cortico-basal ganglia circuit
Proc Natl Acad Sci U S A 111(16): 6063-6068
Group Members
- Joergen Kornfeld